Project Description
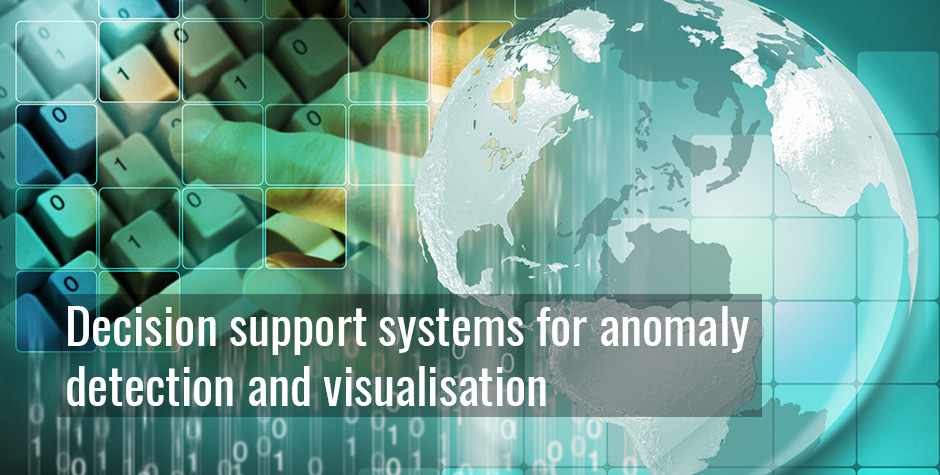
Research questions
RQ1: How does one simulate small transaction systems to capture security important behavior in mobile money based and other telecommunication systems.
RQ2: What are the relevant patterns of money laundering and fraud to simulate and detect.
RQ3: What algorithms are suitable for the detection of these fraud and money laundering scenarios in a timely and effective manner with a sufficiently low false alarm rate given the high volume of data arriving at high speeds.
Aim and scope
To address the problem of a lack of availability of research data (this data is often sensitive for security reasons) we have developed a series of simulators of a retail store that produce security relevant transaction logs. This approach enables us to publish our data for the benefit of other researchers that can repeat and verify our results, and of course, also advance the research based on our results. Using a simulator as a basis for research has other benefits as well. It becomes possible to experiment with environmental factors and investigate how they affect the detection scenario, for example by modifying the nature and level of fraud, scale the background benign transactions to much larger (or smaller) systems, over longer periods of time etc.
Based on partial data from one of our project partners we have built a prototype simulator and applied it to research questions in the area. As far as we can tell we are the first to take this approach, and a as a testament to our success our findings have been very well received. Our first paper publishing this part of our research was awarded the best paper award out of 97 published at one of the largest simulation conferences this autumn (EMSS’13).
The main focus in this project is the question of detection. In a previous KK-project involving the detection of anomalous behavior at sea this approach has shown its merits. It is based on previous research into the detection of computer security intrusions.
Proposed work
Collect and analyze relevant data from our partners. Which includes already stored data in the case of Scorett, and continue to instrument Ericsson’s Mobile Money solution to enable the collection of relevant data from the field. This data will then be the basis of further simulation such that interesting normal and fraudulent behavior can be captured. Then develop and study already existing algorithms for application to our detection scenarios with respect to their performance, how successfully they can be trained both on historical but also streaming data, and how the results of the operation of the detection algorithms can be presented (visually) to the operator to enhance their understanding.
Evaluation/methodology
The evaluation of success is mainly experimental. Having access to a simulator increases the chances of success here as it gives us an unprecedented opportunity to vary scenario parameters to cover and investigate a larger part of the parameter space. This goes for all parts of the research that deals with the understanding of the results of the detector by the operator. When it comes to that, we aim to in addition, also study the feedback from actual users. Our industry partners are uniquely placed here as they have access to actual professional users of these systems which can be called upon to evaluate the relative success of our proposed approaches.
Results so far
In the state of practice today, no really advanced methods for fraud detection are in (at least wide spread) use. Instead simple statistical thresholds and the detection of known patterns of fraud are used. The question arose early in the project: “So how well can these statistical thresholds work?” With a simulator where we can control the environment, level of fraud, and more importantly know exactly which behavior is fraudulent, we were in a position to address that question. The, somewhat surprising result is that empirically statistical thresholds can be set so that we can always cap the loss at a set e.g. percentage of turn over, without incurring an overwhelming amount of false positives. Hence, statistical threshold detection is all that you need in a business setting, esp. the retail fraud setting that we have investigated.
We have also determined that the business owner to e.g. set the thresholds so that an acceptable loss is achieved and so that the investigation of false positives incurs an acceptable cost can use having a simulator available, not only for research etc. but also operationally.
Expected long term results and impact
To find scalable and resource efficient methods to combat fraud, and money laundering (that is a wider and more far reaching problem), and also demonstrate that multi agent based simulation can be fruitfully used in other criminal investigation scenarios.